GMM – Gaussian Mixture Model
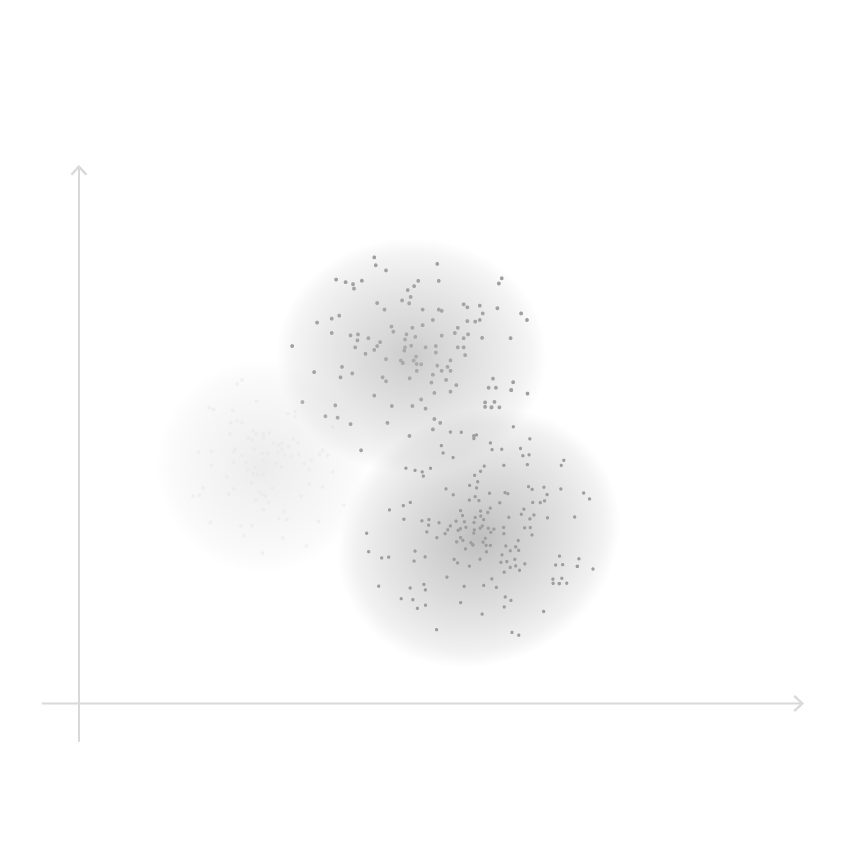
The Gaussian Mixture Model (GMM) stands as a powerful tool in the field of machine learning and data science. At its essence, GMM is a probabilistic model that assumes a dataset is made up of a mixture of several Gaussian distributions. Each Gaussian component represents a distinct cluster or population within the data.
GMM introduces a probabilistic approach to clustering, allowing for the identification of underlying structures within complex datasets. Unlike traditional clustering methods, GMM accommodates data points that may belong to multiple clusters, providing a more nuanced representation of the inherent variability in the data.
GMM finds applications across various domains, from image processing to bioinformatics. Its ability to capture the inherent complexity of data makes it a valuable asset in uncovering hidden structures, whether in customer segmentation, anomaly detection, or, as in our context, fluorescence lifetime analysis.
Fluorescence lifetime distributions often contain a mix of lifetimes from different fluorophores. GMM, in synergy with clustering techniques, aids in dissecting these intricate distributions, allowing researchers to discern and categorize distinct fluorescence lifetime populations within a sample.
FLIM Studio stands out in the complex world of fluorescence lifetime analysis. It smoothly uses the Gaussian Mixture Model (GMM) to enhance your analysis. Drawing on the basic principles of GMM, FLIM STUDIO adds intuitive features to make your analytical experience richer. It allows the user to choose the desired phasor-plot representation, by giving access to a range of phasor segmentation techniques: for example, it is possible to opt for the simple coloring of the phasor cloud or by using a color gradient (see video below).
In the realm of automatic GMM, artificial intelligence takes the reins. The machine learning AI within FLIM Studio adeptly identifies clusters within your phasor plot, assigning vibrant colors for visual clarity. This automatic approach extends further, allowing users to enable/disable the alpha channel, introducing transparency nuances that refine the understanding of overlapping clusters.
For those seeking a personalized touch, FLIM Studio introduces non-automatic GMM. Users can define the number of clusters manually, aligning the analysis with their insights into fluorescence lifetime populations. The flexibility extends to alpha channel settings, providing control over transparency based on the specific demands of the analysis.
Understanding the significance of alpha channel choices is pivotal. Transparency, when set to true, unravels intricate details within overlapping clusters, offering a comprehensive view. On the other hand, opting for false maintains a solid representation, ideal for straightforward interpretations of cluster distributions.
[1] Digman MA, Caiolfa VR, Zamai M, Gratton E, The phasor approach to fluorescence lifetime imaging analysis. Biophys J. 2008 Jan 15;94(2):L14-6.
doi: 10.1529/biophysj.107.120154
Epub 2007 Nov 2. PMID: 17981902; PMCID: PMC2157251
[2] Digmann MA, Gratton E, The phasor approach to fluorescence lifetime imaging: Exploiting phasor linear properties (2012)
https://escholarship.org/uc/item/5g279175
[3] Vallmitjana A, Torrado B, Gratton E, Phasor-based image segmentation: machine learning clustering techniques. Biomed Opt Express.
2021 May 17;12(6):3410-3422.
doi: 10.1364/BOE.422766
PMID: 34221668; PMCID: PMC8221971